
Comminution is simply the size reduction of particles, and its purpose is to liberate valuable minerals from waste prior to concentration. The product is a mixture of particles that contain the valuable mineral (i.e., ore), gangue minerals (i.e., the commercially worthless material that surrounds, or is closely mixed with, the ore mineral), and locked particles/middlings (i.e., particles that contain both ore and gangue minerals). Ultimately, the increased surface area created by comminution produces controlled particle sizes (e.g., blast stemming) and improves recovery (e.g., for chemical reactions).
During comminution, particle size of solid materials is reduced by the application of energy, usually by means of mechanical forces. This is achieved via blasting, primary crushing, secondary crushing, coarse grinding, fine grinding, very fine grinding, and/or superfine grinding.
Getting the setup and design of comminution is so important because the energy costs associated with comminution are often among the largest of all processing costs. It is both an energy intensive and energy inefficient process, typically requiring ~30-50% of total energy used onsite. Consequently, it is essential to characterize and evaluate energy consumption as a function of size reduction.
How do we get our material the right size?
To understand how small your particles need to be ground (and optimize your circuit) requires both knowledge of the mineralogy (easier said than done!) and application of models such as comminution energy laws that relate energy consumption to size reduction. These parameters will help design comminution devices and the processing circuit; and require knowledge of the hardness of the ore (i.e., the ability of ore to withstand breakage and deformation), the tonnage to be processed, and how fine the ore has to be ground.
It is also important to understand where particles break (i.e., their modes of fractures), which can occur at grain boundaries or across grains, depending on the strength and elasticity of the grain boundaries.
Thinking back to our prior geomet posts, there are linkages forming between discussions on deportment and texture now…
How can a geochemist help?
One way in which a geochemist can help is through helping design testwork studies.
Performing metallurgical testwork is expensive, but required. Testwork samples are typically selected based on ore body models that contain a lot of human interpretation, from core logging through to the model generation. The questions postulated below seek a more data-driven approach:
- How can we use a data-driven approach to take into account the variability of an ore body in selecting our metallurgical testwork samples?
- How can we extrapolate the results of these samples throughout the ore body?
To address these questions, a geochemist can organize a scanning XRF or hyperspectral imaging (HSI) study whereby at least a cross-section through the deposit takes into account the variability of the ore body.
Then using the continuous and consistent geochemistry and/or mineralogy and image textural parameters (images are data!) you can domain the ore body. Note that this is not necessarily a geologic or alteration model, but rather a model based on the presence or absence of elements/minerals and textural features.
Metallurgical testwork samples can then be selected from each domain to capture the geochemical and/or mineralogical and textural variability of the ore body.
In response to the second question, the benefit of using a geochemical and/or mineralogical continuous scanning system is that you receive continuous and consistent data over the entire project. Therefore, as long as you do not encounter new lithologies or alteration, you can train a regression model to predict parameters such as a Work Index (Wi; the most common measure of ore hardness) to integrate into an ore body’s geometallurgical model (see example from Carmichael et al., 2019).
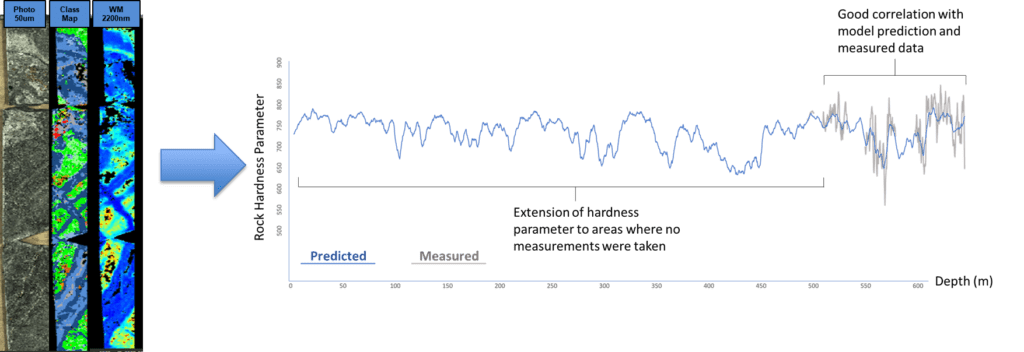
References
- Hunt, J. (2018, May 22-25). Geometallurgy Introduction [Short Course]. Geometallurgy Practical Short Course, University of British Columbia, Vancouver, BC, Canada.
- Klein, B., Wang, F. (2018, May 22-25). Rock Breakage and Ore Hardness [Short Course]. Geometallurgy Practical Short Course, University of British Columbia, Vancouver, BC, Canada.
- Carmichael, T., Scher, S., Crawford, B., Martini, B. (2019, May 20-22). The Predictive Power of Hyperspectral Core Imaging, Applications to Grade and Geometallurgical Parameters [Presentation]. ProExplo 2019, Lima, Peru.